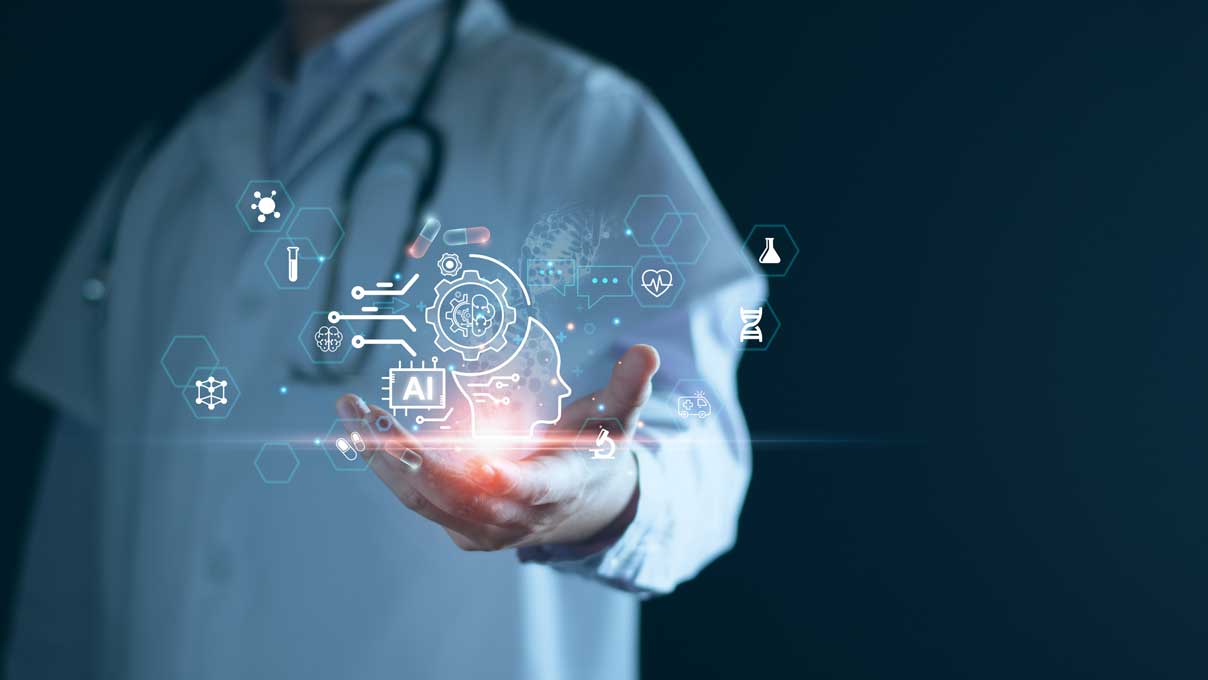
GenAI in Healthcare: How to Stop Hallucinations, Scale Cost Effectively, and Protect Patient Data
Healthcare providers and payers alike have explored GenAI’s applications to improve efficiency and enhance patient care. In a recent Gartner survey, 80% of health system CIOs indicated they’re actively exploring AI use cases. The rate for payers is comparable.
Providers, no stranger to using advanced technology, have used GenAI to improve clinical documentation, brought efficiency to patient messaging, and leveled up revenue cycle management. Payers have reported success with real-time prior authorizations and offering personalized assistance via chatbots. Beyond introducing AI for specific products, contexts, and workflows, business leaders are also deploying AI enterprise-wide to help employees perform at a higher level.
However, this adoption doesn’t come without a cost. Stakeholders are increasingly worried about concerns related to hallucinations, costs, and data protection. With that in mind, I recently hosted 3Pillar’s Chief Innovation Officer, Pankaj Chawla, and Director of Global Innovation, David Evans on the podcast.
This episode is a primer on GenAI for healthcare business leaders. We explored questions healthcare leaders like yourself might have as you consider where and how to deploy GenAI in your organization. For instance:
- How do you guard against hallucinations?
- Is GenAI too expensive to scale across your organization?
- How do you protect patient and company data?
We addressed these considerations and more. Following is a summary of their comments and a roadmap for future action around GenAI in healthcare:
Data Accuracy: Avoiding Hallucinations
Large language models, the engines behind AI writ large, are trained on data at scale. However, this data can produce inaccurate outputs, also known as hallucinations. These models are trained on a predictive basis, meaning they anticipate the next best word or phrase to complete the thought, paragraph, or document. What can follow is called “drift,’ meaning the language model will produce responses that seem plausible but aren’t accurate. Naturally, this raises concerns about whether using AI at scale is practical or useful.
Feeding the large language model with trusted data sources like fee schedules or procedure code ontologies can offer assurance that the AI will base its answers on facts.
By augmenting the AI system with curated data sources, we can have peace of mind knowing the generated responses are reliable and trustworthy. Instead of relying solely on the model’s ability to predict the next best word or phrase, we provide it with inputs that directly relate to the question at hand.
For example, when determining whether an urgent care facility is in-network, the AI should rely on a dataset containing information about the payer’s network. By incorporating this data, we can avoid hallucinations and provide accurate, informative responses.
3Pillars’ Innovation Lab has developed a framework for measuring response quality. This assessment evaluates noise levels and verbosity to ensure the AI application delivers clear and concise responses grounded in accuracy.
Scaling Cost Effectively
The cost of implementing GenAI solutions is a valid concern for decision-makers. Pankaj likens the current state of affairs to the early days of cloud services: Cloud providers are now offering GenAI as a service, similar to how they provide cloud storage or computing power. While the initial cost might be daunting, the long-term benefits of improved efficiency and productivity can make the case for the investment.
David emphasizes cost optimization strategies:
Targeted Audiences: Determine the specific user cohorts that will benefit most from GenAI applications. Avoid deploying solutions across the entire organization if unnecessary.
Internal Hosting: Consider hosting large language models internally to gain more control over costs. Experiment with different models for various use cases. With such infrastructure in place, you can experiment with different AI models to find the best match for specific use cases. This allows you to control costs by avoiding the use of overly powerful models for tasks that do not require them.
Model Flexibility: Design your AI application to allow for easy switching between different large language models based on their performance and cost.
Protecting Patient and Enterprise Data
The recent uptick in healthcare data breaches, like Change Healthcare, has highlighted the critical importance of protecting user and enterprise data. This is only exacerbated by the fact that healthcare organizations are increasingly adopting AI technologies to improve patient care and drive efficiency. At the same time, protecting sensitive patient information should be at the forefront but that’s not necessarily a given.
One of the primary concerns is the potential misuse of patient data to train AI models. There is a risk that confidential information could be inadvertently shared with other parties or used to develop AI applications that could compromise patient privacy. For example, a healthcare organization might use Gemini to analyze patient data, only to find that the model has inadvertently learned sensitive details that could end up in the wrong hands. Not to mention different language processing units (LPUs) have different licensing agreements about whether your data will help train their model.
All of this to say: The sensitive nature of patient data necessitates robust security measures. Our design separates data concerns from language processing. Similar to secure cloud applications, we create private data lakes within walled gardens with firewalls for each client. This ensures patient data remains secure while leveraging the power of GenAI.
Deploy GenAI at Scale with 3Pillar
When evaluating a potential vendor, it’s crucial to carefully review the terms and conditions of the service provider. While some explicitly state that they will not use your data for training purposes, others may reserve the right to do so. Be intentional about opting out of data usage if desired and be mindful of the potential risks associated with accessing these models through the internet.
We’re actively collaborating with healthcare organizations to help them implement GenAI solutions. Our Platform in a Box tool enables rapid deployment of AI technology, making it easy for organizations to explore various use cases. If you’re interested in learning more, please contact us.