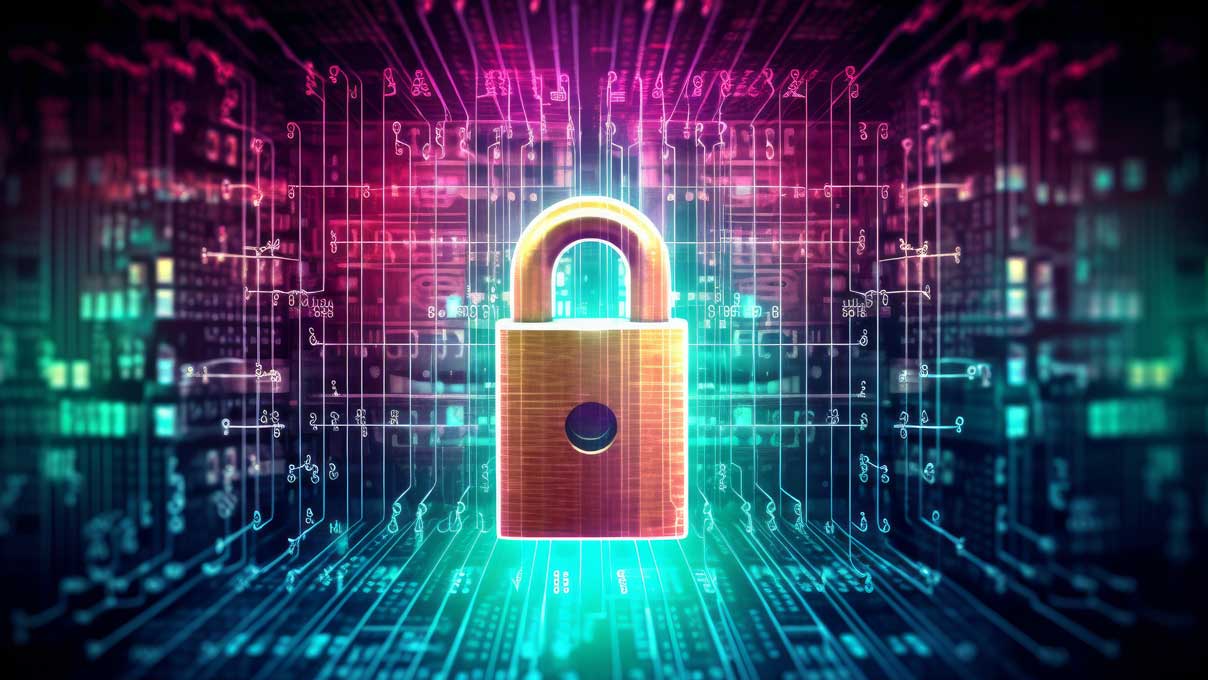
4 Generative AI Risks Every Information Services Company Must Avoid
November 15, 2023
Generative AI now permeates virtually every technology—just look at Microsoft 365 Copilot or new AI-assisted features in LinkedIn.
Information services companies can’t afford to ignore this trend. A wait-and-see approach is highly risky. Those who don’t start investing in AI now will fall behind, when it truly becomes table stakes.
At the same time, jumping into any new technology without a clear plan and vision is equally risky. Tech won’t add value to your data-powered software unless it meets a proven user need.
In other words, AI isn’t a solution. It’s a tool. And if you don’t recognize that, you’ll open your information services company up to four significant areas of risk.
Risk #1: “Wait and see”
Leaders across industries are already adopting generative AI. Business models are already adapting to its capabilities. The time for waiting is over. But how you engage with AI is just as important as engaging in the first place. If you’re just using AI to tinker around the edges and offer incremental value, you’ll miss opportunities and plummet behind more innovative, sophisticated competitors. Generative AI success, then, lies in delivering not incremental, but transformational change:- Accelerating insights generation through increased speed and efficiency
- Enriching answers to complex questions, thereby differentiating the value of your product in highly competitive markets
- Increased revenue generation through upsell, cross-sell, and customer retention through improved user experiences
- Designing and delivering faster workflow solutions for customers, delivering critical insights at the point of need
Risk #2: Wasted resources due to poor product design
Building a bad product is expensive. More expensive than spending a little bit of time and money to ask: will this product or feature actually deliver user and business value? Failing to understand the impact on the user will lead to wasted resources. This is especially true for new, underdeveloped technologies like generative AI. Right now, the precise value generative AI offers users is rapidly evolving, presenting new opportunities and uncertain ROI. Before we spend millions of dollars building an AI-powered product, feature, or experience, it’s incumbent upon you to test those hypotheses. Unless you have a clear idea of where your generative AI capabilities will add value, you’re risking diving deep down a rabbit hole, investing personnel hours and millions of dollars with nothing to show for it. Instead, information services companies should take the same approach to generative AI as they do with virtually every other new technological capability over the past decades:- Conduct user research to deeply understand customers and their needs
- Create a prototype based on informed hypotheses
- Test the prototype with users
- Collect data from tests and invest time in properly interpreting said data
- Adjust the prototype and conduct initial tests if necessary
- Go into production with a product built on informed, tested assumptions
Risk #3: Liability and loss
You can’t unleash an untested technology component onto your business. Not only do you risk wasting resources, but you can open yourself up to dangerous legal liabilities. Although most companies are aware of this risk factor, it’s worth pointing out. Third-party, open generative AI models (like GPT-4, for example) often claim ownership of any data you put into their systems. One of the other major challenges facing companies are risks to data ownership, as the legal protections around data with LLMs and generative AI are currently uncertain. This exposes the business to risk of data laundering and the commodification of customer data by third party providers. As such, private instances of the AI models you use are critical. If you aren’t thinking about your data architecture holistically, you can run into some serious problems. Although your models should have access to the data, not everyone needs that same access. You have to have guardrails, entitlements, and access provisions in place. In other words, the governance framework with which you approach generative AI is just as important, if not more, than mere competence with the technology. Otherwise, you risk serious harm to your intellectual property and your business.Risk #4: Competitive disadvantage
Right now, generative AI is new and emerging. But soon, it will be table stakes. If you don’t want to fall behind, the time to start building AI-readiness is now, not later. However, if you pursue the technology for its own sake, you’ll actually undercut your competitive edge. That’s because technology is rarely a differentiator in itself. It’s how you use the technology to drive value for the user, enhance their experience, and drive business outcomes that makes the difference. This is as true for generative AI as any other emerging technology. Rather than follow the tech-chasers, stand out from the crowd by researching and testing the various applications of generative AI. If you can figure out how to provide value, you’ll put yourself at an actual advantage when all the tech-chasers crash and burn from their mistakes.How to mitigate these generative AI risks
Now that we’ve laid out the risks, let’s briefly discuss how to mitigate them. These strategies fall into three broad categories.Deep understanding of customer needs
Some of the fundamental principles of the Product Mindset are minimizing time to value and solving for need. To understand what “value” and “need” are, you need to first understand your users. You don’t want to build an AI model, think it’s working well, then release it into the wild and have your users break it. You don’t want it giving wrong information because you didn’t test it thoroughly. You must always include the user in building your AI feature or product. A lot of people think they’re really smart on these subjects, but it turns out they’re wrong because they misunderstood the user. Instead, build the prototype, put it into the hands of users, and let them break it. Then figure out what went wrong, iterate, and try again. Once you’re sure you’ve got a product that users enjoy and get value out of, only then are you ready to take it to market.Data quality & readiness
Generative AI rises—and falls—based on the quality of the data used to train it. This means the data must not only be clean and accurate, but stored in a way that’s equally accessible and secure. Otherwise, bad inputs will lead to bad outputs. Information services companies are no strangers to good data practices. You’re experts in wrangling data, creating the right availability of data, and the right velocity of data to make it available for insights generation and advanced analytics. Tactics like building unified data stores are deeply embedded in your DNA. This puts you at a unique advantage when it comes to generative AI success.Data & AI personnel
The people you have on your team and in your corner matter. In addition to insights from the users, there are five broad categories of personnel you must have on your team to avoid the risks mentioned above:- User Research Experts. These people deeply understand users, customer journeys, and customers’ goals, pain points, and jobs to be done.
- AI Product Designer. These people translate an understanding of user needs and goals into AI-powered product experiences.
- AI Product Manager. These people understand the complex interactions of customer needs, business needs, and AI capabilities.
- AI / ML Engineers. It goes without saying that to build an AI-based product, you need someone who gets how AI and ML work.
- Data Scientists. Data quality is key to AI readiness. Fortunately, most information services companies have in-house data scientists who can meet this need.
About the Author
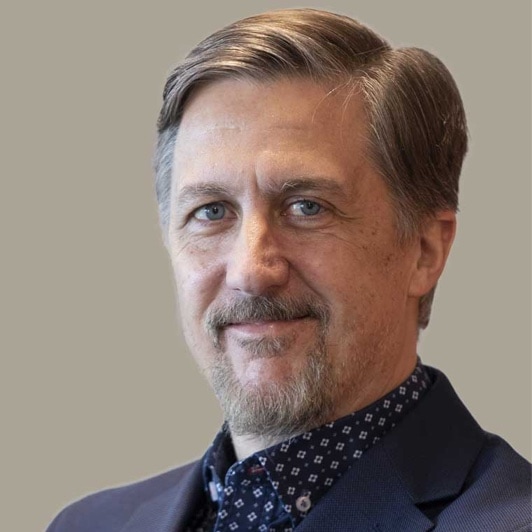
BY
SHARE
Recent blog posts
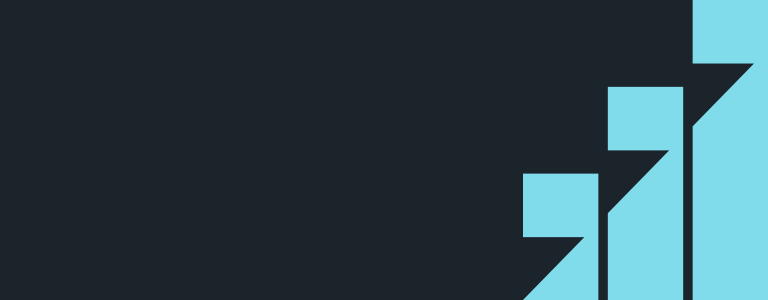
Stay in Touch
Keep your competitive edge – subscribe to our newsletter for updates on emerging software engineering, data and AI, and cloud technology trends.