Improve Business Outcomes Using Analytical Decision Making
Updated: April 20, 2022
Big data has moved from a far-off promise to our new reality. B2B companies now have access to more data than ever—with many getting buried by a deluge of data. But what happens to that information? Do you let it pile up, age, and degrade, or are you using it for analytical decision making? In other words, are you putting it to work?
The thing about data is that there’s this “use it or lose it” element that will make or break your company’s entire strategy, something many brands learned the hard way amid the global pandemic.
As datasets become more complex, organizations struggle to identify relevant insights and which actions represent the best path forward. Even when we’re not dealing with a once-in-a-lifetime crisis, retaining customers and edging out the competition requires analytics-based decision making.
In this article, we’ll examine how big data unlocks opportunity, enables real-time action, and uses data analytics for decision-making.
Importance of Developing a Data-Driven Culture
According to AdWeek, nearly half of all 2020 insights are now irrelevant. While businesses still can’t predict with 100% certainty when the next earthquake, hurricane, or pandemic will strike, the ability to make decisions based on accurate, real-time information allows quick and effective course corrections anytime complications arise.
In a recent article published by Boston Consulting Group, advanced analytics help companies gain the “uncertainty advantage” by addressing three key areas:
- Detection. The ability to analyze a wide range of datasets, assess risks, and uncover opportunities that may seem counterintuitive.
- Multivariate modeling. The ability to use simulations to identify a variety of futures and prepare plans for different scenarios.
- Contingency planning. The ability to anticipate disruption/emergencies and develop contingency plans in advance, including training employees for how to respond to a wide range of potential scenarios.
According to a 2019 Deloitte survey, most executives don’t believe that their company is “data-driven,” and 67% say that they’re not comfortable accessing data or using it to improve decision making in business. Of the 37% of survey participants with the strongest data-driven cultures, nearly half of those companies significantly exceeded their business goals in the past year, making them twice as likely to do so than participants with weak analytics cultures.
In another report, McKinsey researchers found that strategic use of customer behavior data was instrumental in helping organizations outperform their competitors. On average, those companies achieved 85% higher sales growth and 25+% higher gross margins than those struggling to make sense of their data, let alone analyze it to improve decision making.
While there’s no doubt that data-driven decision-making is a critical success factor for many businesses, many aren’t sure how to best leverage the latest tech. How can companies begin using analytical decision making to improve business outcomes? The short answer is, it’s a combination of strategy, tools, talent, and most importantly, culture.
In these next few sections, we’ll walk through the steps toward developing expertise in analytical decision making”
Look at Business Objectives & Prioritize Initiatives Based on Impact
As we’ve mentioned before, making the most out of your data initiatives starts with a laser focus on specific business objectives. Whether your goals are ambitious or easy to achieve, clarity on your goals will simplify the process of understanding the data you want to track, what you want to learn from it, and how you’ll use your findings to improve decision making in your business.
Your goals will be specific to your company. However, common objectives frequently include:
- Understanding consumer behavior
- Driving performance gains
- Reducing indirect expenses
- Managing risk
Keep in mind, even if you plan on targeting multiple objectives, you’ll want to implement and measure each effort separately. Sure, it’s all “big data,” but it doesn’t make sense to measure your fraud detection efforts in the same report as your marketing campaign or operational performance. Analytical decision making is most effective when you are looking at the right numbers in the right context.
With that said, if you’re undertaking multiple initiatives at once, there may be some overlaps in the data you collect. It’s wise to identify what you’ll need up front, so you’re not going back multiple times to regenerate reports.
Locate Relevant Data
Depending on your goal, you can find relevant data in sources like BI platforms, social listening tools, IoT sensors & equipment, CRM software, customer feedback, security logs, surveillance footage, and unstructured text.
By identifying the data that’s available, you can gain a sense of the types of information you can compare to gain insights into your goals. Ultimately, it will aid in improving your company’s analytical decision making.
And, it’s worth considering who is tracking the data, where it lives, and any roadblocks to leveraging it. If much of the data is trapped in departmental silos, how can you partner with other teams to get the data you need? If there are any other obstacles preventing you from using that information, what are they? And, how can you overcome them?
If no data exists, you’ll need to consider what you’ll need to put into place to begin collecting it. Some tools exist to help you fill the gaps, and it’s your job now to identify them. The identification process can also mean looking outside of your organization and incorporating third-party or publicly-available datasets to generate big-picture insights.
Identify Relevant Data
Once you have objectives in place and know what data is available to you, it’s time to get specific. Identify the types of data you need and how far back you need to look to get data that will prove both relevant and sufficient for analytical decision making in your business. As you explore the options, consider questions like:
- Of the data that’s available to you, what could deliver valuable insights?
- If you had a specific type of data, how could you use it to improve decision making?
- What (if any) hypotheses would you like to test—and what data would help you better understand and evaluate each question?
Additionally, consider questions like who, what, when, where, why, and how to help you uncover the types of data you want so you can create a framework for extracting, collecting, and comparing it.
Keep in mind, even if you are asking specific questions and testing specific hypotheses, the types of insights you uncover may surprise you. Findings can lead to significant changes in your business in addition to providing a new lens for analytical decision making.
Build & Test Models
At this stage, you’ll start building models to test your data and answer the business questions you identified earlier. Consider a wide range of models like decision trees, random forest modeling, or data graphs to determine how to best display insights to support users’ goals and answer critical questions.
You’ll also want to collect feedback—another type of data—from the people who will be using these solutions on the job. After all, you’ll want to ensure that end-users have access to tools and insights they can immediately understand and use for data analysis and decision making, regardless of data science experience.
Gartner recommends looking for tools with explainability features, at least for specific functions. Further, Gartner points out that showing users the factors behind an AI-based recommendation can help build trust and encourage adoption among end-users who are skeptical of analytical decision making.
Many analytics platforms offer free trials or guided demos, which allows you to let teams try out various tools to find the right fit. It’s also important to consider the end-user in context. For each use case, consider who is using this solution, what they ask, and what they need to know to improve decision making. Keep in mind you’ll want to avoid presenting more detail than is necessary, because too much data can be as problematic as insufficient information.
Interpret Data Analytics Insights & Draw Conclusions
Once you’ve captured and cleaned the data, you can start analyzing that information to improve decision making for your business. Are the models you’ve selected an effective way to present that data? Do they offer clear next steps for what you should do next? If not, where are you running into information gaps?
Ultimately, the goal here is to eliminate the habit of relying on instinct, experience, or best practices when evaluating the best path forward. “We’ve always done it this way,” prevents your company from innovating to lead your industry. Instead, analytics-based decision making ensures that you and your team can confidently make sound choices.
Put Your Insights to Work
Now that you’ve started analyzing the data, it’s time to establish clearly-defined objectives around changes you want to make, actions to take, and why each matters. It’s also time to define who is responsible for each benchmark and the outcomes you expect.
Define your milestones in clear terms, avoiding goals like “increase revenue,” “be more productive,” and vague deadlines like “it needs to be done by next year.”
Using data analytics to transform your business and improve decision making relies on setting deadlines, measuring progress, and continuously improving your strategy as you gather and analyze new information.
Measure Success
To measure the success of your big data initiative, you’ll first need to revisit your initial data. You’ll want to make sure that the KPIs you’re tracking sync back to the objectives defined in your initial plan. Then, when you pass the deadline for your goal, compare your benchmark to the new data.
What’s changed? How did insights from data analytics impact your business? Data should help you answer critical questions and drive positive change while driving analytical decision making.
Look for areas where decisions led to “wins” and keep an eye out for instances where data led you down the wrong path.
You’ll also want to consider the time analytical decision making takes. If you arrived at the “right” conclusion but took several hours to get there, you might need to refine how you use data analytics to make decisions. Additionally, now is the time to dig deeper into your process by asking:
- Are there issues with the data integrity? If so, how can you solve them?
- If data insights should be presented differently, how can you change this?
- Does your team need improved or different trainings on analytical decision making?
If there’s anything else you can be doing differently, it’s time to use this information to update your strategy to improve business decision making.
Leading with Big Data Analytics
While there’s no shortage of insights hiding in your average corporate dataset, business leaders continue to struggle to leverage data to improve decision-making.
Of course, many of the challenges are cultural, caused by skeptical leadership, departmental silos, and a lack of data literacy across the entire organizational chart.
A recent McKinsey survey found that 61% of business leaders felt that the time spent making decisions was a waste of time.
In a later report, analysts identified three practices to help business leaders improve their decision-making capabilities, based on three different types of decisions. Here’s a quick look at how they broke it down.
- Big bet decisions. Infrequent, high-impact decisions. Big bets come with high-stakes, and decision-making typically stays within the C-suite and stands to shape the company’s future direction. These decisions are broad in scope and may include mergers, acquisitions, and resource allocation.
- Cross-cutting decisions. Cross-cutting decisions, like big bets, tend to focus on the big picture but happen more frequently. These decisions consist of a series of smaller decisions made by interconnected teams. Examples might include improving products, updating brand messaging, or raising prices.
- Delegated decisions. Delegated decisions are smaller in scope than big bets and cross-cutting decisions made by individuals or teams. These decisions typically don’t require input from other departments, such as a sales director updating their team’s training schedule or the HR developing a new recruiting policy.
According to BI-Survey researchers, senior management is the driving force behind big data analytics adoption for improved decision making. A recent survey revealed that in 61% of organizations with integrated big data initiatives, senior management is responsible for organization-wide adoption.
We’ve all seen situations where middle management attempts to spearhead digital transformation efforts within their own department. They might get the green light to adopt new technologies but face barriers when it comes to realizing the full potential of their initiatives. When leadership makes big data a priority and empowers the entire team to use data for analytical decision making, holistic organizational and cultural change is possible. Silos come down, training becomes mandatory, and new data-driven processes gradually replace legacy workflows.
Still, while the top brass must champion initiatives, Amazon’s SVP of HR makes an important point—technology is “everyone’s job.” As such, leaders must think critically about their approach to “cross-functional” and “delegated decisions” that require input from multiple players. We’re beginning to see AI fill in the talent gap, but it’s worth noting there’s a significant learning curve when it comes to using data analytics for effective decision-making.
HBR points toward TD Bank Group’s “Data and Analytics Academy for the Non-Analytics Executive” to bring employees up to speed. The firm offers an immersive training program where participants work through a series of exercises: framing the problem, identifying the data sources needed to address the problem, then applying analytics solutions to find the best path forward.
Data Analytics for Business Decision-Making is Now a Top-Priority
It’s no secret that those companies getting big data and analytics right stand to see a positive impact on the bottom line. As a result, it’s not a question of if data analytics can improve your business’s decision making and transform your trajectory. Instead, the question is, how high can your company soar?
[adinserter name=”Data Analytics CTA”]
Recent blog posts
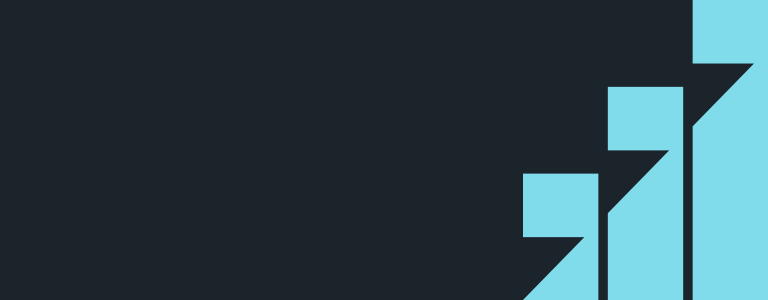
Stay in Touch
Keep your competitive edge – subscribe to our newsletter for updates on emerging software engineering, data and AI, and cloud technology trends.
Thank you for submitting the form