Roadmap for Implementing Big Data Analytics at Your Organization
Brands like Amazon, Facebook, Apple, Google, and Microsoft solidified their tech-giant status early on by using data as a differentiator. These leaders—now among the most powerful companies in the world along with other big names like Spotify, Netflix, Uber, and Airbnb—prove just how unbelievably valuable data can be.
Today, Big Data isn’t just for billion-dollar tech companies or well-funded startups. Small businesses, government agencies, and even legacy manufacturers are ramping up adoption. However, while these technologies are becoming more affordable and accessible, creating a roadmap for a Big Data analytics implementation remains uncharted territory for many brands.
Below, we discuss how to create a framework for data analytics that ensures your program is built on a strong foundation.
Building a Basic Framework for Your Data Analytics Strategy
Before we start talking about creating a Big Data roadmap, there are a few things you need to sort out to ensure you can reliably turn data into actionable insights. While no two roadmaps look the same, every initiative should address these points:
- Define the problem. What problem are you trying to solve? Why are you pursuing this strategy? How will solving the problem impact your business? At this first stage, it’s critical that data science and IT work closely with non-technical users to determine goals and strategies for achieving results.
- Gain a deep data understanding. Once you define a problem, you then need to figure out what data you have and how it can help solve that problem. Figuring out how to use your data to improve customer satisfaction, cut costs, or boost sales should be a joint effort between data scientists and the stakeholders who will benefit from this solution.
- Costs. Keep in mind that implementation costs go well beyond devices and software. If you’re not yet a data-driven organization, you also need to consider the costs of hiring new employees and getting existing ones up-to-speed. After you establish some ideas surrounding costs, compare those numbers against the cost of inaction.
In these next few sections, let’s look at the main components to include in your data strategy—no matter what kind of business you’re in.
Determine What Data You Have and What Data You Need
Consider all of the data types you currently have. That includes everything from user-generated data and website behavior to sales metrics, financials, location data, RFID streams, and other data sources. Which data sets contain insights related to your target use case or the problem you’re trying to solve?
For example, if you’re looking to improve your marketing message, you might audit your system for data that contains information about what customers think about your brand—think NPS surveys, online reviews, and social media mentions.
These voice-of-the-customer data sets can help you understand brand sentiment. You need to pull in data from other resources if you want to truly understand how to create content that speaks to audience needs. You might combine those insights with sales activity, website behavior, and campaign performance.
That way, brands can understand the impact brand sentiment has on sales, as well as which touchpoints moved deals forward or caused customers to look elsewhere. Note that marketing applications require a different mix of data sources than, say, researchers tracking how COVID-19 spreads through communities, or what retailers need for total supply chain visibility.
Identify Gaps and Silos in Your Data Strategy
Once you’ve determined what data you need to gain the insights you’re looking for, find out where it’s stored.
- Is it spread across multiple silos? Here’s the thing: Big Data analytics can only deliver real value when data is both accurate and available to those who need it. An Exasol study found that fragmentation prevents 55% of organizations from getting full value from their data.
- Are there gaps? Once you account for your existing data, look for what’s missing. Are you missing any information that can help you better achieve your main objective?
- What data needs to be integrated? In the marketing example, we mentioned that you might combine social and website analytics with sales data and campaign performance. In that case, you want to make a list of where all critical insights are stored, which sources are already integrated, and which ones need to be connected.
- How will you track progress and determine next steps? What information would allow you to understand the situation in as little time as possible? What information does the end-user need to take action and get the desired results?
It’s also worth pointing out that you probably won’t know what data you need until the effort is well underway. There’s also the possibility that the real root cause of the problem you’re trying to solve isn’t what you were expecting. The point is, you need to build some flexibility into your roadmap so that you can change course as needed.
Evaluate Technical Requirements
Once you establish what data you have, what you need, and a general idea of how that data should fit together, you need to determine the hardware and software tools you need to make it happen. Some of these questions are repeated from the previous section, but they are important to consider in both contexts:
- Address gaps in knowledge. What’s causing the gap? Is it a tools problem or a skills problem? Will you need to add any new data sources? You might consider integrating third-party or publicly-available datasets with internal data for richer insights.
- What data needs to be integrated? Look for solutions designed to unify data from various sources. If your system is a patchwork of legacy equipment, cloud-based apps, and a whole host of devices, it’s important to come up with a plan for getting it all to work together. Again, consider what you’re trying to do.
- Will you need real-time analytics? We recommend looking at technical requirements one use-case at a time. Does the problem you’re trying to solve require continuous availability? Or will you be analyzing insights after the fact? Will you need to act on insights in real-time? For use cases like autonomous vehicles, smart factories, and oil drilling, the tiniest lag could cause significant harm in the real world. If you’re collecting continuous data streams for real-time pattern detection or predictive maintenance, failure probably isn’t a life-or-death situation.However, delays and downtime could prevent you from acting on valuable opportunities or avoiding costly repair jobs.
- Who is the end-user? Sophisticated data scientists or the sales and marketing teams? You want to select tools that fit seamlessly with existing skills and make jobs easier. For example, sales, marketing, finance, and the C-suite need solutions that allow them to size up a situation and act based on their findings. As such, reports should be easy to run and analyze. You may want to look for data visualization tools that allow business experts to make quick decisions instead of forcing dense, text-based reports on busy knowledge workers.
Critical Success Factors Hinge on Organization-Wide Buy-in
To properly leverage data analytics, companies need to become truly insight-driven, which is honestly easier said than done. A 2019 Harvard Business Review study found that 93% of executive participants say people and processes are the main obstacles when it comes to turning raw data into something more meaningful. The report also found that many of those firms are still investing heavily in new tools but failing to address the human element.
While arming your team with the right tools is a start, the best technology won’t do them much good unless the mindset and culture are in the right place. As such, you’ll want to include a few key things in your Big Data analytics roadmap to ensure that your people have everything they need to turn Big Data into a competitive advantage.
Start with a Pilot Program
Don’t launch your data analytics program in one fell swoop. Big Data strategies tend to work best when you start slow.
Start with a pilot program that can help you lock down some clear wins that everyone—from the C suite to the factory floor—can understand. From there, involve everyone in the roll-out and clearly communicate how new initiatives and tools improve processes. Will marketers gain more insight into customer behavior? Can fleet managers avoid costly repairs? Will production teams save time with automated quality testing? The list goes on.
Ensure each team understands what Big Data analytics can do to improve their day-to-day responsibilities, what goalposts they’ll be aiming for, and how to work toward making the proposed improvements. As you develop your data analytics framework, look back at the use-cases that made you consider this strategy in the first place.
Make Room for Adequate Training
Often, companies make the mistake of giving employees a brief tutorial (think vendor demos or a one-day training session) without making sure everyone understands how to use these new tools in the context of their job. According to a Futurum report, employees should possess the following skills.
- Creativity
- Problem-solving
- Ethical judgment
- Curiosity
- Contextualization
While these soft skills don’t guarantee workers have advanced technical skills, focusing on developing them can help employees use self-serve analytics tools in a way that drives real business value.
Implement In Phases
Finally, consider approaching your analytics implementation effort into a series of phased releases. This way, IT leaders can mitigate risks by taking it one capability at a time. This approach helps ensure platform stabilization and can make it easier to introduce new strategies, tools, and processes to employees without overwhelming them, thereby increasing the chances of successful organization-wide adoption.
[adinserter name=”Data Analytics CTA”]
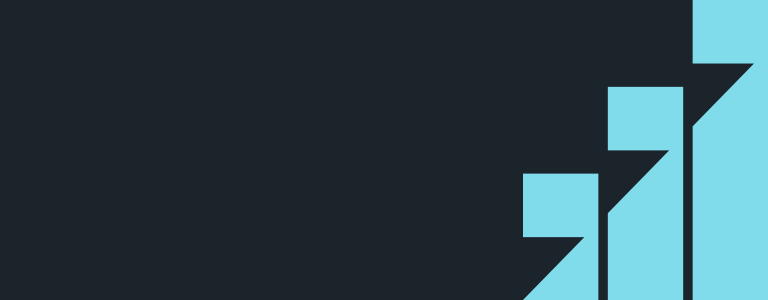
Stay in Touch
Keep your competitive edge – subscribe to our newsletter for updates on emerging software engineering, data and AI, and cloud technology trends.
Thank you for submitting the form