Why Data Analytics is Too Important to Ignore
Sometime around 2013, experts warned that data analytics was “too big” or “too important” to ignore. Countless articles positioned Big Data as a “competitive advantage” and cautioned that those who fail to transform would fail.
Today, Big Data is converging with the Internet of Things (IoT) and advances in AI, machine learning, and self-service advanced analytics tools—which means the benefits promised in the buzzword days are now a reality for leading organizations. However, organizations looking to evolve their data analytics capabilities beyond enhanced reporting must prioritize organization-wide data literacy and invest in the right resources, which could include tools, training, and help from experts on the outside.
In these next few sections, we look at how data analytics can impact businesses in a variety of game-changing use-cases.
The Importance of Data Analytics in Business
If there’s any question as to why data analytics is important, here’s a quick look as to how organizations are already using it to improve business outcomes:
Cost Savings
Many Big Data analytic objectives focus on achieving cost savings across a wide range of business activities. For example, retailers can optimize inventory based on predictions generated from market conditions, social media trends, and weather forecasts. Big Data is also increasingly becoming a critical tool for managing indirect expenses. According to a study by the Dryden Group, cutting indirect costs could save businesses up to 25% of overall costs.
A 2020 McKinsey report points toward the example of a medical technology company that used data visualization and AI to identify and cut indirect third-party spending. The company applied machine-learning and data engineering methods to combine data that was dispersed throughout the IT system and applied cloud-based visualization analytics to identify potential cost savings. The organization could use those insights as leverage during vendor negotiations, saving them 5-10%, depending on the category.
Companies are also using Big Data to stay competitive by focusing on employee retention efforts. Higher retention rates not only reduce the costs associated with training, but also preserve the intellectual capital that allows an organization to remain competitive.
A report by the Harvard Business Review found that more companies are embracing Big Data and machine learning algorithms to predict employee turnover. According to the report, turnover is typically attributed to two main factors:
- Turnover shocks. Think organizational or personal events that prompt employees to make a change—mergers, scandals, new management, or the birth of a child.
- Lack of connection to the workplace. Employees don’t have close relationships with colleagues, or the job doesn’t match their skills/interests/goals.
Researchers sought to improve their ability to predict turnover by gathering publicly available data from articles, the stock market, and ratings from review sites as well as personal data points such as job history, tenure, skills, and education. Recruiters, too, make use of machine learning and data analytics to identify candidates and, ideally, prevent turnover before employees start to get restless.
Efficiency and Productivity Gains
An area that underscores the importance of data analytics is how many different ways these tools can save time: whether that’s improving employee productivity or automating parts of the manufacturing process. For example, logistics companies have long been using Big Data analytics to improve supply chain efficiency and optimize delivery routes. Additionally, Big Data is thought to be the solution to the “last mile delivery problem,” which, by some accounts, can cost nearly a third of the total cost of delivering a package.
UPS is currently using GPS streams from delivery driver phones to track the time between parking the vehicle and dropping off the package. They also use radio frequency identification sensors and GPS to monitor fleets and to integrate live traffic data and weather conditions to optimize routes. Altogether, these data sets inform the big-picture strategy and allow for real-time route updates if a better route becomes available.
In a manufacturing setting, Big Data applications can be used to streamline production processes. For example, Frito-Lay uses machine learning to maintain quality standards during chip production. One application involves using lasers to break chips and uses sound to determine whether they’ve got the texture right. Algorithms then process the chip sounds and use that information to automate quality testing.
Understanding Market Conditions
Other examples of how data analytics can impact business is by allowing companies to understand the trends and economic conditions shaping their industry and to gain insight into how they stack up against the competition. While analysts and data scientists have long used BI tools to perform this type of research, newer innovations in artificial intelligence (AI), natural language processing (NLP), and data processing/analysis are making analytics tools more accessible.
For example, NLP gives non-technical business users an easy way to enter specific queries, much like performing a Google search and receiving an explanation about the insights that allow for quicker decision-making. Additionally, we’re also seeing more tools that offer data visualizations that help users process information faster than traditional reporting tools.
Real-time insights also give organizations the ability to stay one step ahead of the competition. For example, if you’re a retailer and your competitor changes their marketing strategy or lowers prices, you can adapt to those changes as you detect them, helping you maintain—even grow—market share.
Boost Customer Acquisition and Retention
Whether we’re talking about e-commerce, insurance, or SaaS companies, organizations now compete for higher levels of customer satisfaction. As a result, organizations that leverage Big Data analytics to optimize the buyer journey and offer a personalized, post-purchase experience stand to see the best results.
One example is how companies use data analytic platforms for customer behavior and segmentation modeling. This allows them to improve acquisition by mapping behavior outcomes. They can map behavior at every touchpoint in the customer journey and identify which strategies or offers work best and are most effective.
This includes determining where customers are at in the buying process, and which channels they’ve interacted with. And on the retention side, predictive analytics tools help companies provide proactive customer service by using historical data and customer behavior to predict churn, allowing companies to intervene when necessary.
A Driver of Innovation and Product Development
Big Data gives companies the ability to predict what customers want from their products and services. In turn, this data allows companies to get ahead of the competition by bringing innovative products to market faster. Instead of leaning on focus groups and surveys, companies can validate ideas and use multiple data points to create a customer-centric solution. For example, Conagra Brands has used data analytics to track millennial purchasing behaviors in order to inform the direction of innovation in the frozen food category, driving a shift toward convenience and home meal preparation.
Netflix is another example. They use their Big Data-powered recommendation engine to help users find new things to watch. Algorithms are informed by various factors, such as viewing history and what others like them have watched. That same information is applied internally to help the company make decisions about funding new productions. It allows the company to create original content based on what audiences want, thus boosting profitability in the long run.
When implemented correctly, data-driven predictions of which offerings are likely to succeed eliminates the guesswork involved with launching new products. Plus, it avoids costly failures and long trial and error processes.
The Importance of Data Analytics Continues to Grow
The use-cases we presented above represent a small percentage of how companies are creating value with Big Data analytics. Organizations are already leveraging these technologies to attract and retain customers, optimize delivery routes, and create products and services that people want to buy.
That said, it doesn’t matter if you’re a financial services firm, a retailer, or trying to become the next big streaming service, your data strategy must be purpose-driven and focused around big-picture goals. While technologies like AI, machine learning, and Big Data analytics play a critical role in achieving business objectives, they’re just a means to an end. Successful analytics initiatives start with a data-driven culture, cross-departmental alignment, and a laser focus on specific problems and opportunities.
[adinserter name=”Data Analytics CTA”]
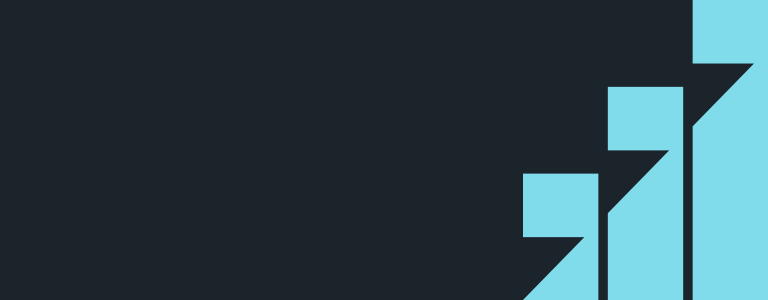
Stay in Touch
Keep your competitive edge – subscribe to our newsletter for updates on emerging software engineering, data and AI, and cloud technology trends.
Thank you for submitting the form