KPIs to Measure ROI from Data Analytics Initiatives
When calculating the return on investment (ROI) for Big Data initiatives, tracking your efforts on a granular level is no easy task. The challenge is, businesses invest in Big Data projects for all kinds of reasons. Many have multiple projects going on at once, each in varying stages of maturity, targeting a completely different set of business problems.
As such, measuring the effectiveness of a data analytics project can’t happen in a single report, nor can you rely on a uniform set of metrics. While key performance indicators (KPIs) are essential for aligning Big Data analytics initiatives to business goals and outcomes, it can be hard to focus on the ones that count.
In this article, we explain how to measure the ROI for Big Data analytics, one use-case at a time.
What Does Success Mean to You?
The first step in calculating the ROI of a data analytics project is to define success within the context of your organization and the use-cases you’re pursuing. Consider all of the ways that data—or, rather, insights—has either directly or indirectly contributed value to your organization.
Think back to your organization’s big picture objectives. Chances are, these efforts span multiple departments and use-cases—each requiring a different set of metrics for tracking its impact. What business problems were they meant to solve? What made you pursue this initiative in the first place?
On a surface level, consider whether internal processes have become easier, faster, or more accurate. For instance, are your data-driven decisions delivering better outcomes? Are social media metrics on the rise? You might start by measuring the impact of those noticeable gains, then move into other areas.
It’s worth noting that KPIs are central to helping organizations unlock the value hiding in Big Data sets. KPIs allow you to prioritize the information that best fits your goals—making it easier on teams to dig deep into relevant data while keeping the “noise” out of the picture.
How Is Data Being Used?
Once you identify your target use-cases and the metrics that represent success, look at how employees are currently using data. How are they leveraging Big Data to improve performance or deliver improved customer outcomes? Where are they falling short? Consider looking at individual performance data to get a sense of whether different analytics, BI tools, or training tactics are needed.
Another big part of measuring the ROI of data analytics is looking closely at all related expenses before digging into the KPIs. Make sure you’re not overspending on areas with little impact on the bottom line (i.e., spending too much to acquire new leads or wasting money on cloud storage meant for smaller data sets).
Becoming a data-driven organization typically requires significant investment in training and upskilling programs. While training comes with the benefit of decreasing employee churn, saving money in the short-term, educating the workforce is expensive. Keep in mind that it may take a long time to see the payoff.
How much have you spent on software, sensors, equipment, and devices to support this initiative? Here, you want to look at everything from monthly SaaS subscriptions to cloud storage and how much you’ve spent on devices and security solutions. While many of these components are essential investments, you might uncover opportunities to cut costs by upgrading or eliminating certain solutions.
Consider the Intangibles
Beyond the direct costs of launching a Big Data initiative, think about the factors that aren’t so easy to measure but are important nonetheless. For example, consider what your business will lose if you put off adoption or your initiative fails. What happens if you’re not the first in your industry to embrace next-gen data analytics? What can machine learning, AI, and natural language processing do to help you lock in the competitive advantage?
Innovation (or a lack thereof) is closely linked to employee retention. Talented employees will likely move on to companies that value innovation and invest in their success. Additionally, not investing in data analytics solutions can put your business at risk.
You might rack up fines due to regulatory non-compliance with CCPA and GDPR, or arrive at work one day and find that your operational equipment has been hacked. Maybe you will fall victim to a data breach and spend days trying to identify and contain the malware responsible for the attack.
On the flip side, you want to evaluate existing solutions for these indirect benefits, too. You might measure employee satisfaction and track retention rates against positive gains over time.
Or, you might get together with your security team to find out how the increased visibility into your network has allowed them to detect more threats faster.
Examples of Data Analytic KPIs Based on Common Use-Cases
In these next few sections, we look at examples of how you might measure the ROI of data analytics, based on what you’re trying to achieve.
Operational Efficiency
In an industrial setting, achieving operational efficiency might mean reducing overtime hours, delivering products to market faster, and streamlining production processes. You might analyze sensor data, overhead, indirect expenses, machine performance, downtime, and environmental conditions. However, operational KPIs might look different depending on whether you’re running the production line or the HR department.
Sample operational KPIs:
- Downtime
- Cost-savings
- Time-to-market
- Overtime hours
- Cycle time
- Maintenance costs
- Production volume
- Capacity utilization
- Number of defects
360-Degree View of the Customer
A 360-degree view provides brands with customer insights from every angle, allowing a nuanced understanding of what motivates customers, how to predict and prevent churn, how to address pain points, and how to deliver personalized, contextual experiences.
Marketers use customer data to experiment with different types of messaging. This allows them to determine which message works best for each segment, which solutions best align with customer interests, and which factors converge to drive the most conversions.
In sales, understanding buyer needs, pains, and motivation allows marketers to adapt solutions and strategies to the individual customer. AI-enabled platforms can calculate propensity to buy and make recommendations based on historical data—thus improving buyer engagement and relationships with existing clients.
In terms of data analytic KPIs, both marketing and sales benefit from the 360-degree view, though each team prioritizes different metrics. Marketing connects performance to campaigns, content, and conversions while Sales is more concerned about close rates and revenue. Still, there’s a lot of overlap, as understanding the buyer is essential for both departments.
Example Marketing and Sales KPIs:
- Net Promoter Score (NPS)
- Number of sales
- Average deal size
- Revenue by campaign
- Revenue by channel
- Churn rate
- Customer lifetime value by segment
- Acquisition and retention costs by segment
Fraud Detection
If we’re talking about bank fraud, organizations often cite faster fraud detection and minimizing their impact as their top goals. Retailers like Amazon, Best Buy, and L.L. Bean use data analytics to take on return fraud.
Sample fraud detection KPIs:
- Chargeback rates
- Cart abandonment rates
- Number of fraud attempts
- Average fraud score
- Order approval rates
Patient Outcomes
In healthcare settings, Big Data analytics is becoming an essential tool for driving systematic improvements to the patient experience. Facilities might look toward Big Data analytics to reduce instances of unnecessary medical procedures, monitor population health, and eliminate billing and prescription errors.
Doctors are also increasingly using predictive analytics as a diagnostic tool. There’s the NIH Precision Medicine Initiative, which helps doctors learn how genetics, lifestyle, and environmental conditions to determine which treatments a patient will respond to best.
The use-cases don’t end there. Here’s a list, which means that within a single hospital system, choosing the right Big Data analytics KPIs is essential for ensuring doctors and admins receive only the most relevant information.
Sample healthcare KPIs:
- Average hospital stay
- Average cost of care
- Hospital readmissions
- Emergency room wait times
- Emergency room costs
- Number of billing errors
- Turnover rates
- Patient safety
- Deaths
Final Thoughts
When done right, data analytics can reveal the exact value Big Data brings to the table and how long it will take before you see a return on investment. Measuring the ROI of your Big Data initiative is more about the business objectives you’re hoping to achieve and the metrics and tools used to measure success, and less about looking at Big Data as a monolith. Data analytics KPIs help companies focus on the right information, so they’re not getting bogged down by security logs, vanity metrics, or the endless noise generated by an ever-growing number of IoT devices.
[adinserter name=”Data Analytics CTA”]
Recent blog posts
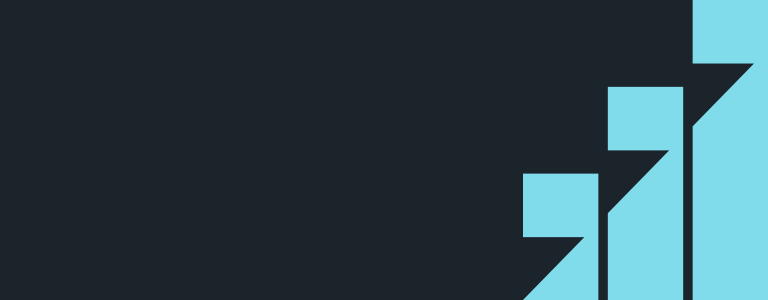
Stay in Touch
Keep your competitive edge – subscribe to our newsletter for updates on emerging software engineering, data and AI, and cloud technology trends.